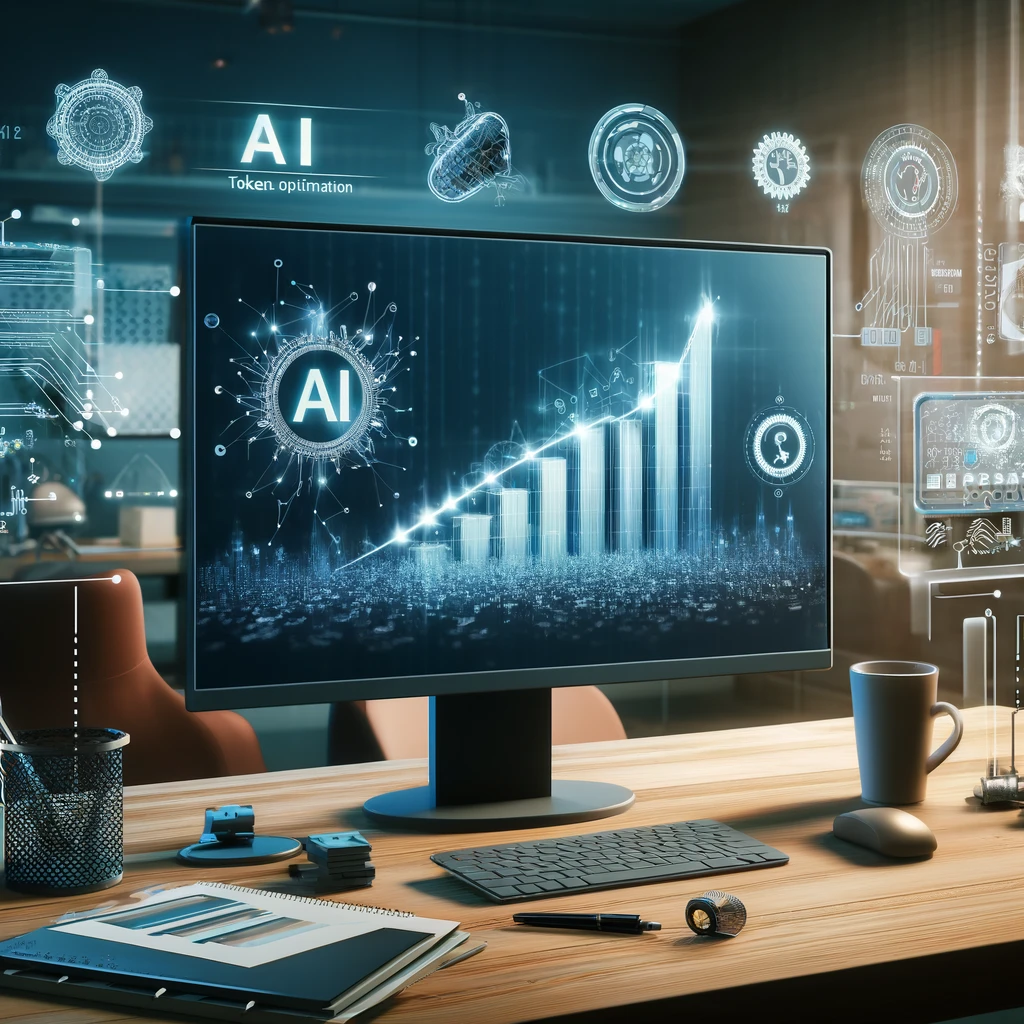
Optimizing GPT API costs: A Developer's Guide to Minimizing API Expenses
Hello, fellow developers! If you’ve been grappling with the high costs of deploying LLMs like GPT-4, GPT-3.5 Turbo, or Claude API, you’re not alone. As we push the boundaries of what’s possible with AI, it’s crucial to keep our wallet in check. That’s why today, we’re diving deep into strategies that not only optimize your usage but also slash those pesky API expenses. Buckle up as we explore the thrilling world of cost-efficient LLM usage, featuring insights from our very own solution at PromptOpti.
Understanding LLM API Costs
The first step to managing your LLM budget is understanding where the costs come from. Each query you send through APIs like the GPT4 or Claude API is measured in tokens, and boy, do those tokens add up! But fear not—efficient prompt engineering is here to save the day (and your budget).
Efficient Prompt Engineering: More Than Just a Buzzword
Efficient prompt engineering isn’t just a fancy term; it’s a necessity. It involves crafting prompts that are concise yet powerful enough to fetch the exact response needed with fewer tokens. For instance, trimming unnecessary verbosity can reduce token usage by up to 30%, significantly lowering costs. Want a practical guide on this? Check out PromptOpti, where we turn the art of prompt optimization into science!
Choosing the Right LLM: A Balancing Act
Not all tasks require the firepower of GPT-4. Sometimes, GPT-3.5 Turbo or even smaller models could do the job just as effectively. Selecting the right LLM for your specific task can lead to substantial savings. It’s all about finding that sweet spot between capability and cost.
Conclusion:
Â